Data-driven water quality modeling
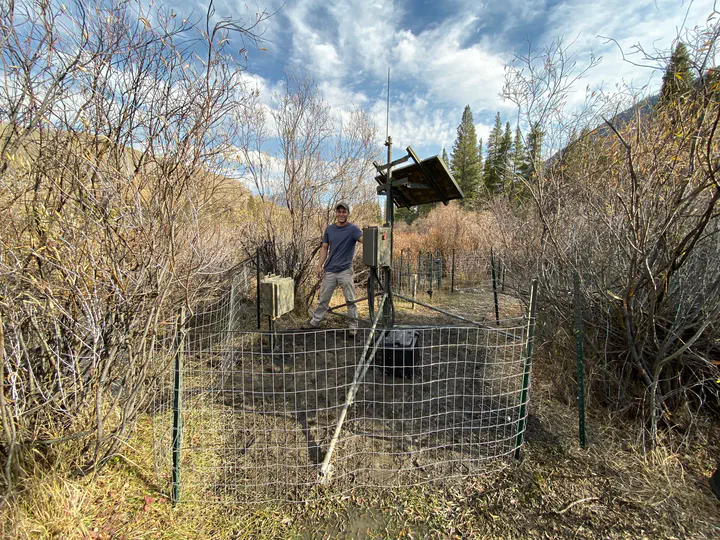
Traditionally, hydrologic modeling has relied on a suite of process-based models developed by many different researchers over years or even decades. These models contain a wealth of domain knowledge about contaminant transport — the physics of water flow and the chemistry of water-rock interactions — but they are relatively fixed and not designed to handle new streams of incoming data; a calibrated reactive transport model, for example, has to be completely re-calibrated if new observations are not in agreement with model predictions.
Recent advances in data science and in situ sensors — when combined with robust biogeochemical models — offer a unique opportunity to address this challenge. We have installed a network of solar-powered environmental sensors at sites in Colorado and Wyoming that provide continuous, high-frequency measurements of hydrologic conditions, microbial metabolic activity and key biogeochemical constituents. Remote connection via cellular modem allows us to access this data and update models in real time.
We are using several techniques to combine the knowledge contained within (process-based) reactive transport models with the flexibility and adaptability of modern machine learning/deep learning models. This includes:
- Assimilating sensor data into a basic reactive transport model with an ensemble Kalman filter
- Training an LSTM with data from reactive transport model simulations, then using transfer learning to apply the model to real-world data
- The same as above, but using sensor data from other sites across the world
- Augementing sensor training data with generative adverarial networks (GAN) then building a GRU, LSTM, or sequence-to-sequence model